Research project Data-driven control and coordination of smart converters for sustainable power system
The shift towards renewable energy comes with technical challenges. We investigate how AI-algorithms can be used in the electric networks of our cities for smart coordination and control of the more volatile, renewable energy resources.
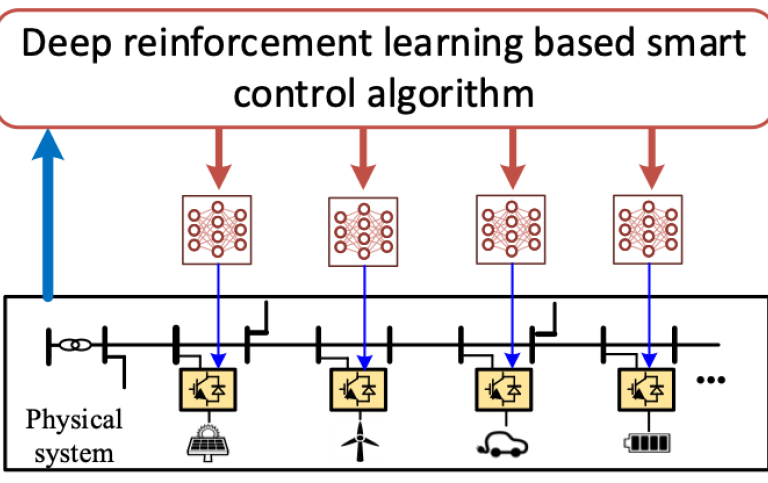
Moving towards sustainability and climate security, electric power systems are going through a major paradigm shift to enable wide integration of distributed energy resources such as solar power, wind power, energy storage, demand response assets, and electric vehicles.
This is a formidable challenge due to the volatility and uncertainty of renewable energy. Today’s grid cannot handle the voltage rise and fast voltage fluctuations from high penetration of renewables, which threaten the security of grid operations and damage electrical equipment. It is widely recognised that the lack of adequate control mechanisms to regulate the voltage within a secure region is a key hindrance of wide integration of renewables into the grid.
Nevertheless, the grid has never been better equipped to handle these challenges than now due to the ongoing digital transformation of the grid, with wide integration of sensors, communications, and advanced data analytics. To meet the full promise of this digital transformation, the technological investments must be coupled with sophisticated control algorithms that can handle the grid’s fast nonlinear dynamics and its stochastic and highly distributed nature.
The goal of this project is to leverage the ongoing digitalization of the grid and state-of-the-art AI approaches to achieve data-driven and communication-efficient control and coordination of smart converters. In particular, our goal is to study how the converters can learn by experience how to make optimal decisions.
We will develop our algorithms in a simulation environment that capture important converter dynamics and interactions. The algorithms will be tested in real experimental microgrids. The developed software will add significant value to industry including advanced reinforcement learning solutions, active grid management, and grid data analytics that have been tested on real hardware. We will make all developments easily accessible for use by practitioners and publicly available.
The full title of this project is "Data-driven control and coordination of smart converters for sustainable power system using deep reinforcement learning".
Project members
Project managers
Sindri Magnússon
Senior lecturer
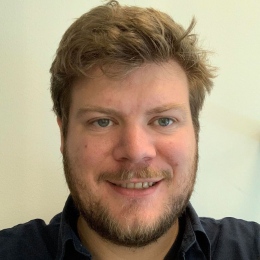
Robert Pilawa-Podgurski
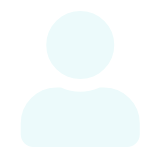